Tackling the AI paradox at the tactical edge
StoryJune 15, 2020
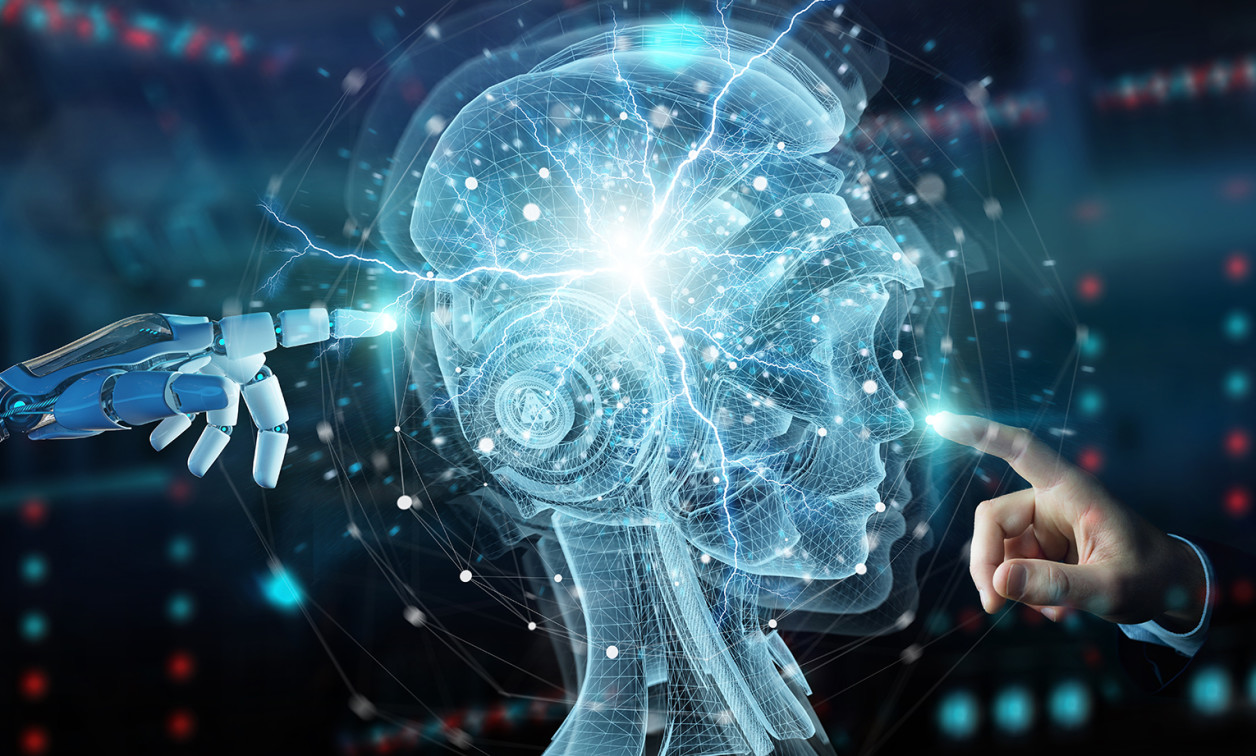
Artificial intelligence (AI), as the general public understands it, is frequently associated with alluring – and sometimes alarming – ideas of talking robots, champion chess-playing computers, and sentient technology humans shouldn’t trust. In the defense industry, however, AI is on the path to becoming a loyal companion to the warfighter and manufacturers alike. As technology progresses, so does the range of defense capabilities that AI could not only supplement, but eventually manage.
Artificial intelligence – which we humans have chummily shortened to AI – is everywhere. Facial recognition on your phone, predictive technology used by Netflix, Amazon’s Alexa: all of these capabilities are powered by machine learning. These technologies aren’t necessarily as enticing as Hollywood makes AI out to be, but they do exert a major influence on the direction of military AI.
The way that the U.S. Department of Defense (DoD) treats both the discussion and utilization of AI depends heavily on the definition of it, which can be subjective. This ties in with what industry professionals have dubbed the AI Paradox: As soon as a capability has been proven to work, it’s no longer considered AI.
Autopilot and fly-by-wire aircraft both use AI but are hardly ever regarded as such. Today, autopilot is thought of as its own capability, with fly-by-wire also its own separate thing. What results is a skewed perception of just how many platforms in the military take advantage of AI and machine learning in day-to-day operations.
What this paradox could also be translated into is simply an integration of AI so seamless that an entirely new capability is now at the military’s disposal. With these advancements, however, come design obstacles that manufacturers face when considering processing power, environment, funding, and the commercial world.
What defines military AI
In order to understand where military AI has been/is going, let’s actually define AI. AI and autonomy, commonly referred to in the same vein, are not quite synonymous. AI is what is needed to enable autonomy, as without it a machine couldn’t become fully autonomous. That being said, AI itself is similarly defined by a specific set of characteristics.
Industry experts maintain that there are four main components that make up an AI system (Figure 1): Situation assessment to understand its environment, the planning loop to decide what goals it wants to achieve and how to handle any tradeoffs, monitoring to make sure everything is being executed correctly, and a learning module that examines prior experience to update the model and avoid shortcomings.
[Figure 1 | U.S. Army Combat Capabilities Development Command graphic depicting the use of AI and human-machine teaming on the battlefield.]
“AI is a system that is interacting with its environment, doing iterative decision-making. It’s reasoning about what it’s seeing, it’s learning what it’s doing so it doesn’t make the same mistakes,” says Karen Haigh, chief technologist at Mercury Systems (Andover, Massachusetts).
While AI is often thought of with futuristic idealization, the reality is that it has been utilized by the DoD for decades, according to Haigh. In 1989, “A Plan for the Application of Artificial Intelligence to DoD Logistics” was published, citing initiatives for AI adoption that began in the late 1950s, even earlier than the plan’s publication.
This 30-year old proposition discussed the concept of predictive maintenance, a capability the military is widely using today. Using AI to bolster military logistics is arguably the most common use of machine learning in modern-day DoD operations. However, this plan also claimed that implementation would be a done deal by 1992; this long-gone date highlights the gradual nature of AI acquisition in the military.
The best way to map out the history of military AI and its growth in an easily digestible way is to line it up with commercial AI advancements. Commercial AI is booming because capabilities powered by AI already exist in data-rich, significantly less-contested environments than that of theaters of war. Therefore, military AI has required more time and engineering capacity to widely adopt.
“We’re in an interesting situation in terms of the transposition of who’s leading the tech,” says Tammy Carter, senior product manager at Curtiss-Wright (Ashburn, Virginia) “You can see that now when graphics processing units (GPUs) come out and they’re available in the commercial world for at least a year, maybe two, before they get into the embedded space because they have to be hardened, et cetera. So right now, we’re a generation behind for most GPUs because of the ruggedness of them.”
The current state of military AI
Dr. Tien Pham, chief scientist of the computational and information sciences directorate, U.S. Army Combat Capabilities Development Command Army Research Laboratory, asserts that military AI is and has always been driven by the commercial sector. This claim ties right back into the advantage that commercial AI has by existing in environments with generous amounts of data available for use.
Predictive analysis using open-source intelligence is an AI-powered capability that has proven successful in the commercial sector because of the data-rich environments. Could it be just as beneficial for defense if military-relevant data wasn’t so scarce? While this situation presents an engineering obstacle, it in no way has halted progress:
“We can see increasing innovations in algorithms and computing resources for AI in complex data environments, AI for resource-constrained processing at the point-of-need, AI with limited training data, and generalizable and predictable AI,” Pham says.
Predictive maintenance is finally starting to make the strides that the DoD hoped it would in 1992. Intended to replace scheduled maintenance – a process that ensures all aspects of a platform are being routinely serviced but often results in costly sometimes unnecessary labor – predictive maintenance would streamline the control loop. Switching from schedule-based maintenance to AI-powered, condition-based maintenance could very well be implemented in the near future.
“With machine learning, you can start doing things like putting sensors on platforms like vibration sensors that would tell you how much the rotor is vibrating on the platform, and too much vibration would tell you that something may not be in good shape,” says David Jedynak, chief technology officer at Curtiss-Wright (Austin, Texas). “Then you could take those sensors and train a machine learning-based format to know what a good helicopter looks like from a sensor standpoint and maybe even train it to know what some faults look like.” (Figure 2.)
[Figure 2 | Curtiss-Wright’s family of rugged mission computers support GPU acceleration (and some also deep learning acceleration) to empower embedded machine learning capabilities. Shown is the Jetson TX2i-based Parvus DuraCOR 312.]
Saving time, money, and lives is the goal of the greater adoption of military AI. Whether an unmanned ground vehicle (UGV) executing a beyond-line-of-sight operation to detect a possible improvised explosive device (IED), or an algorithm that could analyze an image and detect something a human couldn’t, military AI manufacturers hope to use this technology to better augment military procedures. Standing in the way of this progress, say manufacturers, is adequate processing power and the means to achieve it.
Where military AI hopes to go
Collecting large amounts of rich data is something that AI has proven to do well, but one barrier to wider adoption is the processing power required to transmit that data in an efficient way.
“When you’re collecting imagery, video, or large swaths of the electromagnetic spectrum, you want to be able to do something with that as quickly as possible,” says Shaun McQuaid, director of product management at Mercury Systems (Figure 3). “That’s where I think having the capability to deploy these kinds of AI and machine learning applications on the platform comes into play. Without that capability, you don’t have the ability to do anything with those large data sets in real time. That, I think, is the advantage that the modern architectures that support machine learning and AI platforms bring to the folks who are utilizing them.”
[Figure 3 | The composable data center (cloud) is comprised of powerful scalable CPUs, GPGPU coprocessors (both now with AI hardware acceleration), fast storage, wideband fabrics (PCIe), and I/O. Mercury aims to mirror this architecture for embedding into applications with our OpenVPX high performance embedded edge computing (HPEEC) solution like the SFM6126.]
Additionally, understanding this processing power from a size, weight, power, and cost (SWaP-C) standpoint is just as important. Integrating machine learning capabilities onto a small unmanned aerial system (UAS), for example, requires a level of embedded computing expertise cognizant of the type of technology being put onto the platform, power consumption, and wattage among other factors.
“The algorithms are usually trained on the bigger boxes because there’s so much data and you need so much processing to learn the algorithm,” Carter says. “But when you’re actually going to implement it, you take the algorithm you developed, or the code and the programs, translate, and cut it down so it can actually run on these smaller devices.”
Stitching – or persistent intelligence, surveillance, and reconnaissance (ISR) where signal-processing and AI technologies are pulled together and joined – is another machine learning capability that military AI manufacturers are trying to sharpen. According to Haigh, there remains a lot of data that the military isn’t processing terribly effectively.
“We can do sonar data, radio frequency data, or image data, but we are not terribly good at stitching those concepts together,” Haigh says. “For example, say you had a counter-UAS system at an airport, can you stitch together the radio signals and radar signals along with a camera image from all around and not just from the central station? Can you stitch all of that together to gather if there is an actual drone threat at that airport? We don’t have that stitching yet, but we’ll get there. Every year we take another step up the ladder in terms of the complexity and richness of the data we can pull together.”
What may stand in the way is the procurement process for AI services, which is arduous. Currently, in order for the Pentagon’s Joint Artificial Intelligence Center (JAIC) to begin the process of accelerating AI adoption and deployment, it must go through contract vehicles offered by various DoD organizations. On this subject, Lt. Gen. Jack Shanahan, director of the JAIC, has argued that the procurement process should become unilateral to quicken acquisitions.
The various approaches used in the procurement process is partially responsible for the gradual adoption of AI in the military, and may also partially explain why the AI that has been fielded generally executes lower-level tasks. The money isn’t quite where it needs to be to take bigger risks in development of AI technology, but manufacturers are hopeful that things will soon start to change.
Funding the future
“In the wake of the COVID-19 crisis, there is an expectation that defense budgets will be impacted globally,” says Amir Husain, founder and CEO of Spark Cognition (Austin, Texas). “As a consequence, force levels could potentially decline, and therefore, there is a real need to maintain effectiveness and capability as these [AI] trends unfold. Autonomy is one of the key innovative technologies that will be at the heart of the future force, not only due to competitive reasons but now also because of budget concerns.”
The DoD’s Third Offset Strategy, promulgated in 2014, has played a noteworthy role in the military’s switch from putting a monetary emphasis on legacy hardware like planes, ships, and tanks to concentrating on a more software-defined battlefield. The internal motivation spawns from a basic need to keep up with adversaries like China, and eventually develop a semblance of what Lt. Gen. Shanahan termed an “enterprise cloud solution.”
“AI is thought of as a product, with talk of AI-specific budgets. This makes sense for research projects, but not for the acquisition of production-level capability,” Husain says. “When you acquire a capability, you do so because it is better than legacy capabilities, or competitive alternatives. Saying we need to devote a small amount of money to ‘AI-only’ acquisition is like saying we need to allocate a sum of money to ‘math-only’ or ‘physics-only’ acquisition.”
The progress, innovation, and DoD acknowledgement seem to be there. Selling AI as a concept is easy – the buzzword certainly is attention-grabbing – but pitching a nonphysical algorithm can be tricky. However, it’s becoming increasingly more difficult to ignore just how pivotal AI will be for defense technology.
“DARPA [Defense Advanced Research Project Agency] and the JAIC have both received increased funding,” says John Hogan, director at BAE Systems Fast Labs (Lexington, Massachusetts). “And they have cast a wide net beyond traditional government contractors to ensure they capture innovation from the entire community.”