AI, open standards to play key role in future SWaP designs for UAS payloads
StoryMarch 17, 2023
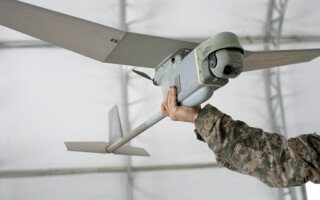
The uncrewed aerial system (UAS) industry faces constant pressure to reduce the size, weight, and power (SWaP) requirements for the payloads they carry. Those pressures often conflict with the mounting data-gathering and bandwidth demands of these systems. Open standards – along with artificial intelligence (AI) and advanced machine learning (ML) technologies – will be key to development in the military UAS arena.
Regardless of whether they operate on the ground, in the air, or at sea, many autonomous system payloads act as sensor platforms for intelligence, surveillance, and reconnaissance (ISR) and other applications. ISR requirements call for more and more capability in the same footprint, placing immense pressure on sensor system designers to reduce the size, weight, and power (SWaP) footprint for the payloads.
Suppliers currently are pushing the limits due to the increased rate of change in the market, as component size and power increases dramatically – meaning increases in heat – which makes it harder to fit everything into a typical 3U form factor, let alone cool them, says Mark Littlefield, senior manager of embedded computing products and system solutions at Elma Electronic (Fremont, California).
Also impacting these designs is the “rapid increases in interboard and system I/O [input/output] communications performance, and the speed of adoption of these high-performance protocols,” he adds. “The density of processing and high-bandwidth pipes between modules is really what is enabling the autonomy.”
Engineers at Omnetics (Minneapolis, Minnesota) – which builds connectors for use on spacecraft and UAS platforms – have seen this trend in the satellite market as well. “You’re getting to the point where you’re looking at putting more in a smaller form factor,” says Scott Unzen, the company’s director of marketing and market development. “The ability to do that and meet shock, vibration, and G forces is harder and harder.” (Figure 1.)
[Figure 1 | Examples of two Omnetics Nano-D connectors, which are designed for small-form-factor applications in extreme environments. (Photo courtesy Omnetics)]
When it comes to satellites [and autonomous systems], there has been exponential growth in capabilities such as geopositioning, vision systems, and data transfer. All of this means more data, Unzen says. “The biggest thing they’re looking for is data throughput,” he says.
This demand for data throughput creates challenges must be met by the industry. Dan Mor, director of the video and GPGPU [general-purpose graphics processing units] product line at Aitech Systems (Chatsworth, California), says SWaP constraints require creative solutions to satisfy continually rising data needs.
“Companies must operate within strict constraints when it comes to SWaP, despite the reality of users having a huge amount of data to process due to high-resolution cameras and high-bandwidth raw data,” Mor says.
“This means that we need to find high-performance processing units with low power consumption,” he adds. “The ability to process big amounts of data with a high PPW [performance-per-watt] push is by using a parallel-computing architecture in order to offload calculation from the CPU to [artificial intelligence] and parallel-enabled GPU architecture.”
The primary task for Mor’s team is to ruggedize and cool down high-performance units in a very small envelope and keep them running at full performance, he says. To do this, a company must address all aspects: hardware, software, and mechanical design. that is where open standards come in – the open approach makes it easier for companies to address SWaP in a holistic fashion.
MOSA and SOSA have changed the game
The migration of reduced-SWaP design to open standards as derived from the Modular Open Systems Approach (MOSA) and the Sensor Open Systems Architecture (SOSA) has changed the industry substantially, with companies focusing more on designing products within the context of a defined architecture rather than creating proprietary, closed solutions, Littlefield says.
Ultimately, it is in a supplier’s best interest to follow these standards rather than go the proprietary route, he argues.
“The most successful component suppliers and system integrators are those who embrace the standard and leverage the tools and strengths that it provides,” Littlefield says. “Fighting the standard, or trying to circumvent it, may give a marginal benefit, but is detrimental to overall ease of system integration and makes eventual technology insertions far more difficult. That change in focus from ‘doing things my own way’ to ‘doing things within a defined architecture’ is already having a real impact on our community.”
[Figure 2 | Elma Electronic’s family of high-speed SOSA aligned backplanes are intended to help accelerate development of a common, modular architecture across critical C5ISR and EW systems.]
Littlefield notes that not only has he noticed more requirements for SOSA in payload and control systems, but he has also come across people asking for SOSA alignment, even though they may not actually require it. He adds that SOSA has become a value-add for customers that results in easier integration and long-term maintenance.
Mor has also noticed a shift toward SOSA conformance in the industry: “SOSA means interoperability, and the ability for the integrator to quickly replace any system board from one vendor to another,” he says. “This interoperability creates tight competition between rugged-boards providers and pushes them to develop competitive advantages to differentiate them from other boards manufacturers.
“It’s becoming a ‘must-have’ for many projects,” he adds.
SOSA isn’t the only source of open standards getting increased attention in an industry focused on improving efficiency and lowering costs in the area of reduced-SWaP designs. Littlefield says he has seen interest in integrating safety-critical aspects like ISO 26262 into MOSA and SOSA – although he notes that there is nothing concrete in this area yet.
“It’s clear that people are starting to think about applying SOSA to the problem of vehicle autonomous control, and perhaps even leveraging common computing and sensing resources for both the autonomy and mission-sensing problems,” he says. “It’s early days, though, and it’s not clear if that trend will continue – there are good reasons to keep those two domains separate.”
AI and ML enable reduced SWaP
Open standards are just one part of the equation. To achieve further gains in reducing SWaP, the industry is increasingly turning to artificial intelligence (AI) and machine-learning (ML) technology to help drive more performance despite the reduced power.
With the continuing development of new, advanced threats and growing geopolitical tensions (the war in Ukraine being a prime example), edge computing using AI and communications technologies will gain traction in the market moving forward, Mor asserts.
“Platforms need not only high-performance, SWaP-optimized systems for image and video processing at the edge, but also AI that handles object recognition, classification, and tracking and networking for deterministic communication and real-time decision-making,” he says. (Figure 3.)
[Figure 3 | The Aitech U-C5300 SOSA aligned AI graphics board is aimed at use in AI delivery, video analytics, image processing, and other battlefield applications.]
The electro-optical sensors and cameras mounted on an uncrewed aerial system (UAS) or ground vehicle can capture real-time footage from the battlefield, enabling warfighters to zoom in on specific areas of interest. From there, SWaP-optimized embedded computing systems using AI edge-computing capabilities could send the video footage to the warfighter, perform the processing and analytics, and then send a decision to the ground-control monitor, Mor says.
Specifically, this AI at the edge could help a warfighter identify an object of interest, classify it, locate it, extract coordinates, and then send those coordinates to a control or remote station. Such tools thereby enable warfighters in the field to make what Mor calls “quick and precise” decisions that are critical on the battlefield. (Figure 3.)
ML is also enabling reduced SWaP; while AI is connected to ML, they describe different things. AI is when a computer is able to learn and problem-solve much like the human brain, whereas ML is a subset of AI that involves using mathematical models to learn without direct inputs from a human. Basically, ML is a method that AI uses to increase its own intelligence.
ML presents even more opportunities for the industry to increase the utility of reduced-SWaP systems, Littlefield says.
“ML is real, and the community is hungry for products that enable or improve the performance of ML,” he says.
“It’s not so much a case of ML improving SWaP, but more that the kind of hardware that enables ML is now available within the SWaP parameters companies like Elma tend to work with,” Littlefield continues. “For example, a few years ago ML would have taken a 400-watt GPU to operate, which isn’t practical for a VPX-based electronic warfare (EW) or signals-intelligence system deploying a pod off the wing of a UAS. Now, however, there are GPUs and processors that perform ML calculations within the SWaP constraints of a 3U or 6U conduction-cooled VPX card.
“Thus we are seeing ML being applied to just about every sensor modality and application domain that our industry serves, and products being fielded to serve those applications,” he adds.