Why space needs artificial intelligence
StoryJune 17, 2020
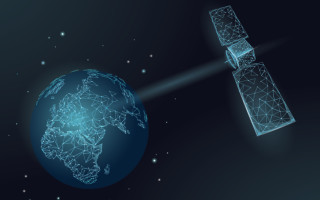
By Paul Armijo and George Williams
The modern-day revolution in artificial intelligence (AI) is fueled by neural networks, a concept that dates back to the 1950s; this concept has surged in the last decade under its new, much improved, guise called deep learning. Deep learning is empowering systems with unrivaled abilities to perceive their environments visually and to make sense of human language through voice or text. But what do face recognition in family photos or customer-service chatbots have to do with advanced space tech and military intelligence-gathering? Quite a bit, it turns out, and the common denominator is data.
[GSI Technology graphic.]
Deep learning can consume massive amounts of data and distill all of it into compact machine learning models. When deployed, these trained models can be used for a myriad of tasks here on Earth: object recognition, language understanding, predictive analytics, even complex decision-making. In space, these models can perform similar roles as they process massive amounts of sensor data to gather and interpret intelligence, predict mission-critical events, facilitate human-computer interaction, and empower local-vehicle autonomy.
From Earth to Mars … and beyond
As artificial intelligence (AI) is added to missions, more and more Earth observational (EO) data will be processed on board instead of sent down to Earth. This evolution toward increased AI will take many years. In the meantime, thousands of satellites will continue beaming data back to the surface until end of life. This reality means that we still need to build advanced high-performance computing (HPC) systems here on the ground that use smarter, power-efficient algorithms to crunch all that data. We will need AI to process multispectral imagery not just over space, but over time. This kind of analysis is critical for improved weather forecasting and real-time disaster awareness, as well as just-in-time first response. We can leverage AI not only to protect people against natural disasters, but also against human-made ones. A tremendous amount of space junk is hovering right above us, and we can use AI to predict when and where the most dangerous ones will make landfall.
Vehicles that operate at the topmost layer of the atmosphere – known as pseudo-satellites – take the form of high-altitude balloons or drones. They have a variety of uses: military intelligence-gathering, maritime monitoring and surveillance, environmental observation, climate prediction, and border patrol.
These vehicles collect a huge amount of heterogeneous data, so there is a pressing need to fuse all this raw data and process it all on board. AI can help in this instance, thus avoiding costly transmission of data to the ground. These pseudo-satellites can remain airborne for long periods of time, running on solar energy. Giving these vehicles the ability to make navigation decisions locally could be key for completing even longer-term missions autonomously.
At low Earth orbit (LEO), we start to encounter our first real satellites. LEO is a very busy place these days: A record number of small satellites have been launched in the last few years at low orbit, with many more on their way. With thousands of satellites at nearly the same altitude and little traffic control, we will need AI to predict possible collisions and to enact appropriate countermeasures. The newer small satellite “constellations” are tightly interconnected in order to service a new global internet; all of these will need highly adaptive AI-based algorithms to reliably route traffic within this highly challenging topology. At LEO, we also encounter the first humans who will live in space for long periods of time. AI has a clear role to play in this realm as personal assistants: not just robotics, but as emotionally aware companions for the long haul.
As LEO fills up, new satellites will start to populate the medium Earth orbit (MEO) lanes. AI will be needed at this distance for collision avoidance and autonomous countermeasures. The cost of transmitting sensor data at MEO becomes even more expensive in terms of power and time, so local AI-based data processing is even more important at this distance. At geosynchronous Earth orbit (GEO), on-board processing is critical. The satellites at this distance not only observe Earth activity but also watch solar flare activity as well as cosmic-ray events such as gamma-ray bursts. Because these phenomena can be dangerous to activities here on Earth, improving the detection and recognition capabilities through AI will improve the related early-warning systems.
Deleterious climate change on Earth has been linked to the aggressive depletion and consumption of natural resources. The moon seems an incredibly large untapped resource, but it remains unknown precisely what resources lie underneath its dusty surface and where those resources live. AI has been used here on Earth to predict and locate underground reserves of oil and minerals, and it will be an invaluable tool for doing the same on the moon. The continued exploration of the moon needs to scale beyond current efforts, which now require roundtrip communications to the Earth. New exploratory missions will utilize swarms of autonomous drones that will require AI-based sensor processing and local navigation decision-making.
Vehicles en route to Mars must be quite sophisticated to be completely autonomous. The trip is dangerous and future missions will require immediate adaptation to potential impairments, such as solar and cosmic radiation bursts and even object collisions. AI could be employed to not only predict such events, but also to make emergency corrective navigational maneuvers.
The exploration of Mars, much like that of the moon, cannot scale given the current approach – slow-moving rovers that are predominantly remote-controlled from the Earth. The new army of proposed exploratory drones is autonomous and AI-driven; they are intended to navigate on their own, balancing risk-versus-reward decisions at every turn. When humans eventually make their homes on Mars, their environments must be completely controlled by smart algorithms that control every aspect of life support.
As missions move beyond Mars to other planets, and beyond the solar system, there must be a balance of storing data and transmitting it back to Earth in the right amounts. Onboard data processing will help, but data will also need to be compressed aggressively between transmission windows. AI-based compression has been shown to be effective and could be also used in the far reaches of space where it is needed the most.
Going forward
The modern-day democratization of AI and machine learning has sparked nothing short of a revolution. Organizations everywhere are rethinking their product and research strategy at all levels of the technology stack – from silicon to software to applications. The one-two punch of both open research and open source has created an unprecedented availability of these state-of-the AI algorithms, and new hardware that now accelerates AI has become a commodity. That is the situation here on Earth. As we go farther from the planet’s surface, the varied radiation-imbued environments create significant challenges for commercial off-the-shelf (COTS) hardware, necessitating highly custom implementations.
The goal: To create modular and cost-effective computing systems for all space-related efforts, from ground-based HPC data centers to deep-solar-system exploration missions. We are committed to the open nature of AI and we are welcoming collaborators to join this effort bringing novel computing architectures to mission-critical systems in space. The GSI Technology project is called FRACTALS [Fault tolerant and Resilient Associative Computing for Artificial inteLligence in Space] and has already announced its first partner, SHREC (NSF Center for Space, High-Performance, and Resilient Computing).
Paul Armijo is the Director of Aerospace & Defense Business Sector at GSI Technology. Paul has had the privilege of leading numerous flagship programs and technology development efforts over his career to further enable the space community. Paul received his B.S. in electrical engineering from Arizona State University. He may be reached at [email protected].
George Williams is Director of Computing and Data Science at GSI Technology. He’s held senior leadership roles in software, data science, and research. He is an author on several research papers in computer vision and deep learning. Readers may reach him at [email protected].
GSI Technology
www.gsitechnology.com