DARPA aims to develop defenses to thwart attempts to deceive machine learning algorithms
NewsFebruary 08, 2019
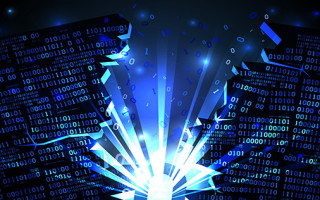
ARLINGTON, Va. Officials at the Defense Advanced Research Projects Agency (DARPA) created the Guaranteeing AI Robustness against Deception (GARD) program, which aims to develop a new generation of defenses against adversarial deception attacks on machine learning (ML) models.
Current defense efforts were designed to protect against specific, pre-defined adversarial attacks and, remained vulnerable to attacks outside their design parameters when tested. GARD seeks to approach ML defense differently – by developing broad-based defenses that address the numerous possible attacks in a given scenario.
“Over the last decade, researchers have focused on realizing practical ML capable of accomplishing real-world tasks and making them more efficient,” said Dr. Hava Siegelmann, program manager in DARPA’s Information Innovation Office (I2O). “We’re already benefitting from that work, and rapidly incorporating ML into a number of enterprises. But, in a very real way, we’ve rushed ahead, paying little attention to vulnerabilities inherent in ML platforms – particularly in terms of altering, corrupting or deceiving these systems.”
“There is a critical need for ML defense as the technology is increasingly incorporated into some of our most critical infrastructure. The GARD program seeks to prevent the chaos that could ensue in the near future when attack methodologies, now in their infancy, have matured to a more destructive level. We must ensure ML is safe and incapable of being deceived,” stated Siegelmann.
GARD’s novel response to adversarial AI will focus on three main objectives:
- The development of theoretical foundations for defensible ML and a lexicon of new defense mechanisms based on them;
- The creation and testing of defensible systems in a diverse range of settings; and
- The construction of a new testbed for characterizing ML defensibility relative to threat scenarios.
Through these interdependent program elements, GARD aims to create deception-resistant ML technologies with stringent criteria for evaluating their robustness.
GARD will explore many research directions for potential defenses, including biology. “The kind of broad scenario-based defense we’re looking to generate can be seen, for example, in the immune system, which identifies attacks, wins and remembers the attack to create a more effective response during future engagements,” said Siegelmann.
GARD will work on addressing present needs, but is keeping future challenges in mind as well. The program will initially concentrate on state-of-the-art image-based ML, then progress to video, audio, and more complex systems – including multi-sensor and multi-modality variations. It will also seek to address ML capable of predictions, decisions, and adapting during its lifetime.