CAML program aims to improve human/machine partnerships
NewsFebruary 01, 2019
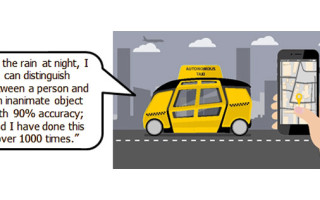
ARLINGTON, Va. The Defense Advanced Research Projects Agency (DARPA) launched the Competency-Aware Machine Learning (CAML) program, which aims to develop machine learning systems that continuously assess their own performance in time-critical, dynamic situations and communicate that information to human team-members in an easily understood format.
When it comes to forming effective teams of humans and autonomous systems, humans need timely and accurate insights about their machine partners’ skills, experience, and reliability to trust them in dynamic environments. At present, autonomous systems cannot provide real-time feedback when changing conditions such as weather or lighting cause their competency to fluctuate. The machines’ lack of awareness of their own competence and their inability to communicate it to their human partners reduces trust and undermines team effectiveness.
“If the machine can say, ‘I do well in these conditions, but I don’t have a lot of experience in those conditions,’ that will allow a better human-machine teaming,” says Jiangying Zhou, a program manager in DARPA’s Defense Sciences Office. “The partner then can make a more informed choice.”
That dynamic would support a force-multiplying effect, since the human would know the capabilities of his or her machine partners at all times and could employ them efficiently and effectively. In contrast, Zhou noted the challenge with state-of-the-art autonomous systems, which cannot assess or communicate their competence in rapidly changing situations.
“Under what conditions do you let the machine do its job? Under what conditions should you put supervision on it? Which assets, or combination of assets, are best for your task? These are the kinds of questions CAML systems would be able to answer,” she says.
Using a simplified example involving autonomous car technology, Zhou described how valuable CAML technology could be to a rider trying to decide which of two self-driving vehicles would be better suited for driving at night in the rain. The first vehicle might communicate that at night in the rain it knows if it is seeing a person or an inanimate object with 90 percent accuracy, and that it has completed the task more than 1,000 times.
The second vehicle might communicate that it can distinguish between a person and an inanimate object at night in the rain with 99 percent accuracy, but has performed the task less than 100 times. Equipped with this information, the rider could make an informed decision about which vehicle to use.
DARPA has scheduled a pre-recorded webcast CAML Proposers Day for potential proposers on February 20, 2019. Details are available at: https://go.usa.gov/xE9aQ.
The CAML program seeks expertise in machine learning, artificial intelligence, pattern recognition, knowledge representation and reasoning, autonomous system modeling, human-machine interface, and cognitive computing. To maximize the pool of innovative proposal concepts, DARPA strongly encourages participation by non-traditional proposers, including small businesses, academic and research institutions, and first-time Government contractors.
DARPA anticipates posting a CAML Broad Agency Announcement solicitation to the Federal Business Opportunities website in mid-February 2019.