Optimizing AI-transportable compute architectures
BlogJuly 30, 2021
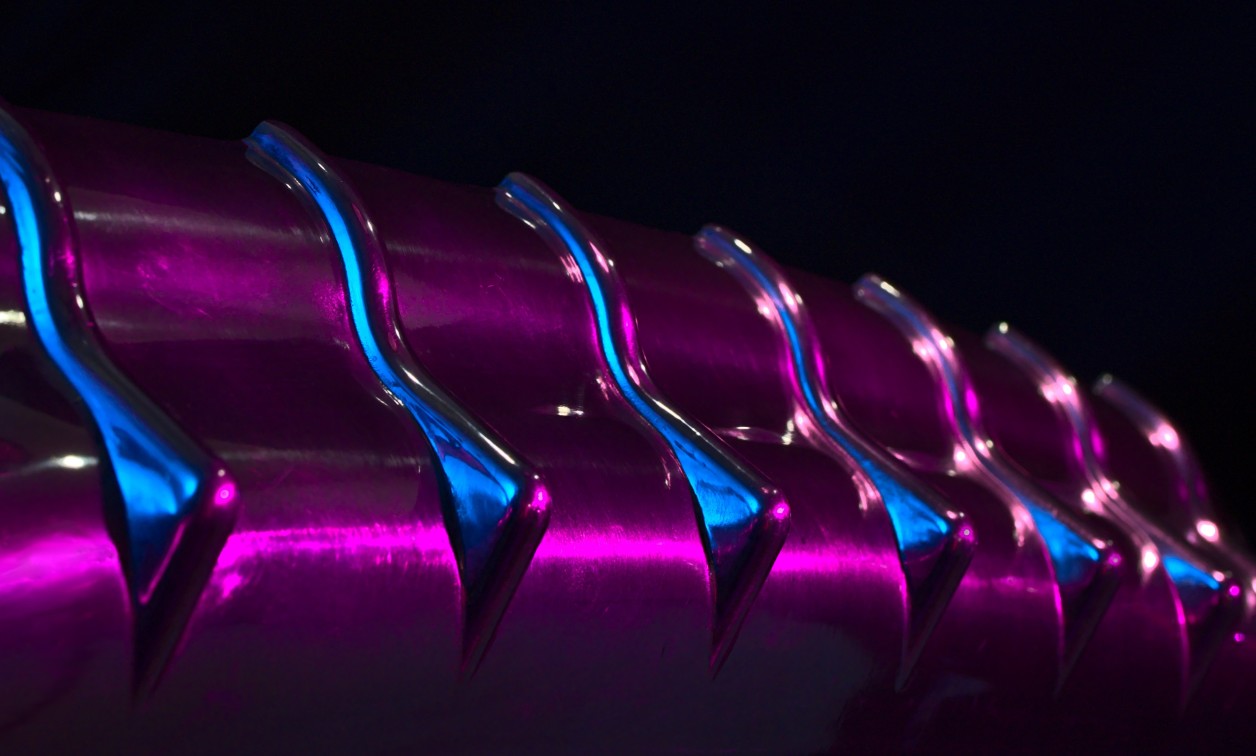
By Braden Cooper, One Stop Systems
Artificial intelligence (AI) in the military electronics industry is growing at a surreal rate. Recent innovations in various fields have coincided to bring the most powerful advancements in computing, sensor technology, and software to mission-critical scenarios. Just as GPUs continue to outpace Moore’s law in terms of raw compute power, new sensor and networking interfaces bring larger and larger data sets in need of computing. These new technologies provide a key opportunity to bring the power of commercial and scientific AI advancements to military-transportable installations. The primary distinctions (and obstacles) between civilian data center-type AI applications and military-transportable deployments are the environmental, power, and security requirements of the missions.
One clear example of the need for AI system deployment at the edge is threat detection in military terrestrial, air-borne, or marine vehicles. Like civilian self-driving-car object identification, military threat-detection systems are capturing incoming sensor data, feeding the data to a pre-trained AI model, and inferencing indication of threats within the sensor data. This workflow, while only a few steps, requires several different complex hardware layers. The sensors deliver a stream of data to the compute nodes, which in turn distribute the actionable intelligence to the proper subsystems, all of which operate on a framework of high-speed storage and interconnectivity.
Building the hardware architectural map becomes an exercise in optimizing the continuous data throughput with the size, weight, and power (SWaP) restrictions of the vehicle. While this workflow can be optimized in a civilian data center by adding another rack of computer systems, most military vehicles’ unique power, form factors, and environmental conditions make the challenge and need for optimized AI transportable systems apparent.
A possible solution to the challenges of this workflow is to broadcast the sensor data stream to a remote or mobile data center, which can support the less edge-optimized computer systems; thereby eliminating the need to ruggedize AI compute nodes. However, as the volume of data grows, the communication path between sensor data storage and the “cloud” or remote data center quickly becomes the throughput bottleneck. To take full advantage of the latest AI technology in military-transportable AI applications, edge-optimized converged systems should be used that integrate the full workflow.
These military rugged systems maximize the sensor data ingest rate and match it with the compute, storage, and networking speeds throughout. In this no-bottleneck architecture, the balanced dataflow can meet the compute needs of the data in a scalable manner that can grow as the data grows. Eliminating the need to compute remotely means sensor data can be captured, processed, and used for real-time inferencing and decision making.
Breaking the dependency on remote data centers and cloud computing optimizes the throughput of the AI workflow, but does come with new challenges. As with all electronics systems in military vehicles, the converged AI systems must be designed to meet the rigid MIL-STD environmental conditions as well as the unique power-delivery systems of their respective vehicles. Commercial off-the-shelf (COTS) servers built for data centers have the luxury of operating in air-conditioned rooms with 220VAC, single-phase power. To truly optimize the AI workflow in military vehicles that often operate in less-than-ideal conditions, the systems hosting the AI building blocks must be designed, tested, and qualified to meet the stringent requirements of the missions they will go on to support.
Braden Cooper is a product marketing manager at One Stop Systems.