Cognitive electronic warfare: Countering threats posed by adaptive radars
StoryJanuary 31, 2017
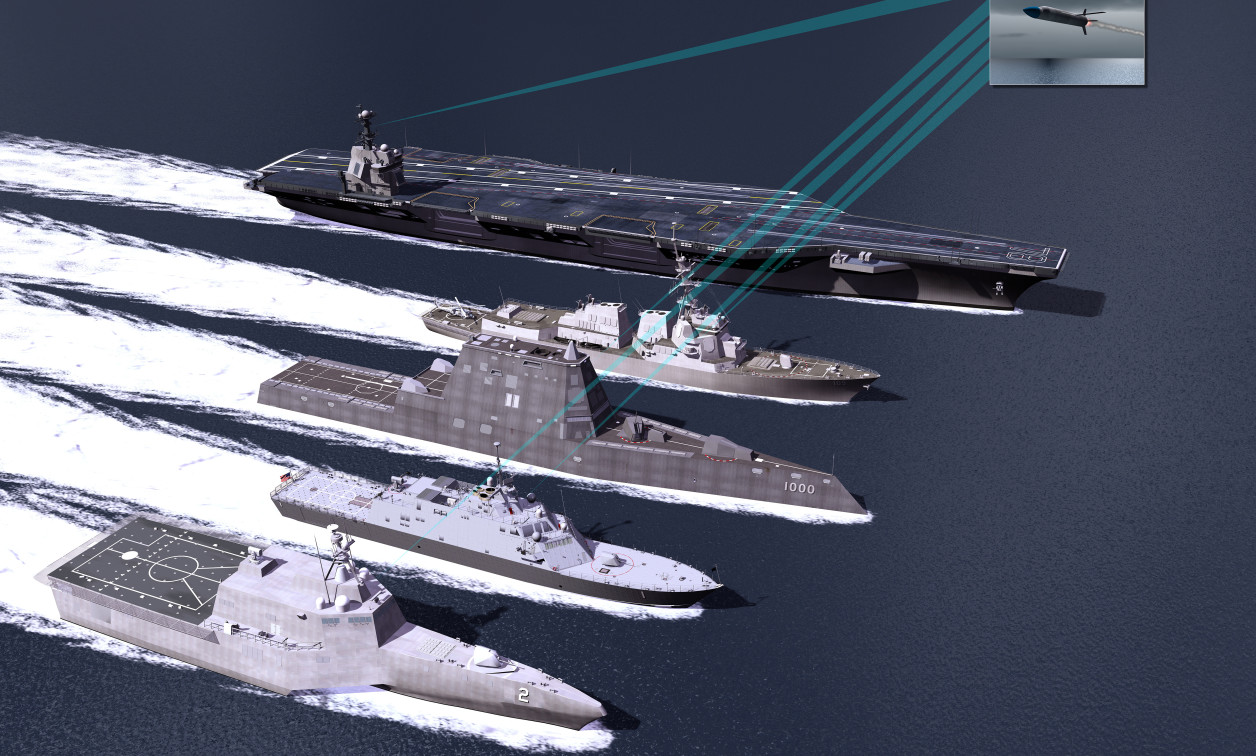
Threats posed by adaptive radars to electronic warfare systems are a colossal challenge for the U.S. Navy, but a combo of advanced signal processing, intelligent algorithms, and machine learning techniques are being developed to help warfighters detect and counter them.
Electronic warfare (EW) systems – whether on land or aboard U.S. military ships and aircraft – tap the electromagnetic spectrum to sense, protect, and communicate. But, when necessary, these same systems can be turned against adversaries to deny their ability to disrupt or use radio, infrared, or radar signals.
Today’s EW systems tend to rely on databases of known threats with predefined countermeasures, which can limit their ability to quickly adapt and respond to new advanced threats. Soon, these systems may increasingly be tasked with isolating unknown hostile radar signals within dense electromagnetic environments and responding quickly with effective electronic countermeasures.
Threats from adaptive radars aren’t necessarily new, according to Joe Ottaviano, director, Electronic Warfare, for Lockheed Martin (www.lockheedmartin.com), but are challenging for EW systems to detect.
Years ago, back when the U.S. Navy’s Surface Electronic Warfare Improvement Program (SEWIP) Block 1, 2, and 3 were formed, “it was a key requirement to be able to detect and counter adaptive radars to provide warfighters with the best information about the radar and its mission,” Ottaviano explains. “We need to do this while performing our mission – before the enemy knows we’re there. Block 2 is designed to deal with adaptive radars.”
BAE Systems (www.baesystems.com), another defense contractor working within the realm of adaptive radars, was recently awarded a $13.3 million contract by the U.S. Defense Advanced Research Projects Agency (DARPA) to extend its work on the Adaptive Radar Countermeasures (ARC) project, with the goal of enabling airborne EW systems to counter new, unknown, and adaptive radars in real time.
Cognitive EW technologies developed by BAE Systems for the ARC project rely on advanced signal processing, intelligent algorithms, and machine-learning techniques.
Adaptive radar vs. “unknown” radar
Adaptive radars, for starters, shouldn’t be confused with “unknown radars,” both of which cognitive EW addresses, as John Tranquilli, technical director for signals and communications processing at BAE Systems, points out.
Both radar types do pose challenges for EW systems and warfighters, albeit with differences. “For the class of unknown radars, current systems rely on a database of threat knowledge to compare observed characteristics to a list of known radars,” Tranquilli explains. “When a radar comes up as unknown or is misclassified as some other radar – because it’s either a really new radar or an existing one that’s behaving outside prior known bounds – today’s systems have very limited ability to characterize the threat or turn around an appropriate response.”
Radars are currently evolving away from fixed analog systems – which aren’t easy to add new capabilities to – toward digitally programmable variants that can easily add or change to unknown behaviors with agile waveform characteristics. “This challenge will increase the prevalence of ‘unknown radars’ and lead to truly adaptive radars,” Tranquilli continues.
Adaptive radars, which pose an even greater challenge, can sense their environment and design transmission characteristics on the fly to maximize radar performance. “This allows a radar to have new waveforms – or new pulse processing – for every transmission, whether it’s improving target resolution or mitigating interference effects,” Tranquilli says.
The objective of cognitive EW “is to enable EW systems to autonomously characterize these threats, automatically generate effective countermeasures against them, and monitor the effectiveness of our countermeasures so we can adapt our techniques if they’re either ineffective or if an adaptive radar attempts to adapt around our responses,” he adds.
Recognizing and countering adaptive radars
How complex is recognizing and countering hostile adaptive radars? It falls under the category of “very complex.”
For SEWIP, a significant amount of software was developed as part of Block 2 to specifically address these challenges. “While development is a challenge, testing is becoming even more of a challenge,” Ottaviano notes. “Defining what’s effective and how effective the detection and response are requires a tremendous effort. We’re working with our Navy partners to continue to improve how we define success within a highly adaptive environment … and right now it’s our biggest challenge.”
From BAE Systems’ perspective, “adaptive radars challenge our ability to isolate the pulses from threatening radars from other hostile, friendly, and neutral signals, our ability to deduce the threat posed by the radars, and our ability to select and configure an appropriate response to achieve our desired effect,” Tranquilli says. “A host of adaptive and machine-learning algorithms are necessary because these challenges are too complex to design a simple series of rules around.”
Role of machine learning and artificial intelligence
What role are machine learning and artificial intelligence (AI) playing in adaptive radars? There’s already a “fair amount of intelligence built into the new operational systems such as SEWIP,” Ottaviano says.
Is there a role for a fully autonomous system? “It depends on the application,” Ottaviano notes. “I can easily see small packages being fully autonomous within a five-year window. For systems that deal with weapons, you can view it as another tool within the toolbox to allow warfighters to own the electromagnetic battlespace.”
For its part, AI can be “a loaded term,” Tranquilli cautions. “Typically, it refers to systems that attempt to have algorithms replace the functions a human would perform in an operation,” he adds.
But Tranquilli thinks there’s likely “a place for AI in the near future of cognitive EW, because as threat environments become more contested and complex, it can help reduce the mental load on pilots and planners.” This is “autonomy,” a field in which BAE Systems is an active player.
Machine-learning techniques, on the other hand, “involve data-driven processing that allows a system to not be beholden to a static set of features, databases, or rules,” Tranquilli points out. “I can’t speak to the level of adaptation and autonomy allowed by today’s systems, but as threat systems continue to advance they’ll adapt more quickly and change their behavior faster … so algorithms and decision engines that can outpace our adversaries are key to future success. This is the central tenant of Bob Work’s Third Offset Strategy – pursuing next-gen technologies and concepts – and is funding advances within the cognitive EW arena.”
Future cognitive EW
Interestingly, in terms of the hardware involved, Lockheed Martin is finding that a commercial off-the-shelf (COTS) open-architecture approach allows a rapid insertion of new capability to continually improve the ability and performance of the SEWIP Block 2 system.
“These systems can and have been upgraded in an immediate fashion while underway to continue to provide the warfighter with new tools,” Ottaviano says. “We’ve seen a resurgence of this capability now beyond the surface EW community … even moving into decoy and off-board platforms. This provides a quick time to solution because the approaches we’re taking aren’t hardware specific and don’t require long development cycles.”
Tranquilli sees a place for advanced hardware in the future of cognitive EW “because systems will need faster response times, more prevalent RF and spatial coverage, and broader sets of available responses,” he explains. “The great thing about cognitive EW is that it can be a scalable capability so portions of the technology can be implemented into systems that exist today, while advances in the hardware, firmware, and software resources will unlock the ability to get more power out of the technology.”
Lockheed Martin is also currently working on a new U.S. Navy development contract to provide MH-60 helicopters with enhanced EW surveillance and countermeasure capabilities against anti-ship missile threats. (Figure 1.)
Figure 1: A U.S. Navy MH-60R Seahawk helicopter attached to Helicopter Maritime Strike Squadron (HSM) 74 lands on the flight deck of the aircraft carrier USS Harry S. Truman (CVN 75). (DoD photo by Mass Communication Specialist 2nd Class Lyle H. Wilkie III, U.S. Navy/Released)].
(Click graphic to zoom)
As part of it, they’ve developed a system – known as the Advanced Off-Board Electronic Warfare Active Mission Payload (AOEW AMP AN/ALQ-248) – that is essentially a self-contained EW pod hosted by an MH-60R or MH-60S to provide the Navy with advanced anti-ship missile detection and response capabilities. It’s designed to work independently or with the ship’s onboard electronic surveillance sensor, SEWIP Block 2 AN/SLQ-32(V)6, to detect incoming missiles and evaluate where they’re going. AOEW then uses RF countermeasures to deter any incoming missiles.
“Our system will help create a coordinated attack against these threats to keep our warfighters safe by controlling the electromagnetic spectrum and disrupting adversaries,” Ottaviano says.
Sidebar | Basic cognitive EW concepts
The basic concepts of cognitive electronic warfare (EW) fall into one of three categories: signal analysis and characterization, countermeasure response design, and countermeasure effectiveness assessment, according to BAE Systems’ John Tranquilli, technical director for signals and communications processing.
Without giving away specific algorithm approaches used for any given cognitive EW program, Tranquilli notes that “the ‘advanced signal processing’ BAE refers to typically consists of approaches that generate, train, and adapt features and models to characterize the behavior of a threat, design a response based on a mix of expert a priori knowledge and online observations of effectiveness, and use our models to infer the impact of our techniques based on the physics of what a radar must do to accomplish its task as opposed to what our techniques must do to deny them success.”
Beyond DARPA’s ARC program, BAE Systems is working with DARPA on other projects to bring advanced “adaptive” algorithms to the RF domain. This includes the Communications in Extreme RF Spectrum Conditions program, the Cognitive Spectrum Sensing component of the Computational Leverage Against Surveillance Systems program, and others.
During the past decade, his group – Signals and Communications Processing, within the Technology Solutions Business Area of BAE Systems’ Electronic Systems – has focused on “bringing the combination of domain knowledge and adaptive algorithm expertise needed to architect and implement these concepts for existing and future electronic systems,” Tranquilli says.